Breast cancer GWAS studies show reliable genetic association in over 200 distinct genetic regions1. The linkage disequilibrium in these regions may complicate pinpointing the true causal variant(s) in each of these regions, in particular for those regions that do not contain directly protein coding genes. In order to address this challenge, we apply a machine learning approach. This allows us to compare the regulatory properties of breast cancer associated alleles to the alleles that do not increase the risk for breast cancer.
Adapted from Chen et al.2 who developed the artificial intelligence (AI) tool “Sei”. This AI learns to predict regulatory properties of any human sequence. We apply this to elucidate potential functional variants for breast cancer associated regions from GWAS studies1. A) A graphical representation (UMAP) for the clustering of 30 million individual human genome sequences, clustered by their predicted cell-specific regulatory properties. Clusters for specific types of regulatory regions (e.g. promoters in lower right cluster) as well as cell-specific regulatory sequences (right hand list of sequences) are identified. Highlighted region exemplifies how genetic variation may cause shifts in predicted regulatory properties in pane B and C. B) A genetic variant in the human genome changes a sequence, the variation of the T-to-C nucleotide is entered in the AI-based prediction. C) The genetic variation depicted in B causes a profound change in the predicted regulatory properties of the specific sequence due to the change. In this example, the reference allele - T- gives the sequence a predicted stem cell enhancer property, whereas the sequence formed by the alternative allele -C- falls in the low signal sequences. Such regulatory shifts may explain the association of specific genetic variants and mutations to the occurrence of breast cancer.
Whilst uncovering which variants convey the risk for breast cancer is a step forward, it is important to consider the variants or genes that are associated to breast cancer in their biological networks. Analyses that focus on how individual variants may act in concert in their genetic networks may uncover more biological processes involved in breast cancer risk. Here we apply an analysis strategy similar to a successful study into the autoimmune disease multiple sclerosis3.
Schematic overview of our biological network analyses A) Breast cancer-associated genetic variants (SNPs) are obtained from the latest large-scale genetic screens for breast cancer1. B) Per associated SNP, all variants in high LD are obtained through analysis against large-scale reference panels. C) Predicted tissue specific QTL properties are obtained and validated. D) Publicly available protein-protein databases provide a per-tissue overview of which pathways have most evidence for involvement based on the predicted functionally relevant SNPs in an analyses performed in multiple sclerosis in a similar style3 E) A per-patient genotype profile is used to populate the biological networks identified in an individual-specific biological network analysis. This individual, tissue-specific scores can be used in further analyses of the individuals’ phenotypic properties such as disease course, treatment response and recurrence rate.
The presence of breast cancer in any first-degree female relative in general nearly doubles the risk for a proband and the risk gradually increases with the number of affected relatives. Current advances in molecular oncology and oncogenetics may enable the identification of high-risk individuals with breast-cancer predisposition. The best-known forms of hereditary breast cancer (HBC) are caused by mutations in the high-penetrance genes BRCA1 and BRCA2. Other genes, including PTEN, TP53, STK11/LKB1, CDH1, PALB2, CHEK2, ATM, MRE11, RAD50, NBS1, BRIP1, FANCA, FANCC, FANCM, RAD51, RAD51B, RAD51C, RAD51D, and XRCC2 have been described as high- or moderate-penetrance breast cancer-susceptibility genes. The majority of breast cancer-susceptibility genes code for tumor suppressor proteins that are involved in critical processes of DNA repair pathways. This is of particular importance for those women who, due to their increased risk of breast cancer, may be subjected to more frequent screening but due to their repair deficiency might be at the risk of developing radiation-induced malignancies. It has been proven that cancers arising from the most frequent BRCA1 gene mutation carriers differ significantly from the sporadic disease of age-matched controls in their histopathological appearances and molecular characteristics.
Figure 1. Data mining and analysis workflow. (A) A total of 583 SNPs in 203 candidate genes from the ROS metabolizing and signaling pathway were selected from an initial pool of 4,000 SNPs in 233 genes. These 583 selected SNPs were analyzed for associations to 3,351 mRNA transcripts from a whole-genome expression analysis, filtered for signal quality (ratio of spot intensity over background exceeding 1.5 in at least 80% of the experiments in each dye channel). A subset of SNPs and a subset of transcripts that belong to biclusters were identified. (B) A heat map of −log10 (P value) of SNP–transcript associations, with range from 0 to −log10(9.5E-005) = 4.02. Bright yellow indicates significant associations. Rows and columns are reordered to highlight biclusters, subsets of SNPs, and transcripts that share significantly many common significant associations (one example is highlighted with a red oval). (C) GO analysis was used to study the overrepresentation of GO functional classes in these sets of mRNA transcripts. The size of the corresponding node of the GO tree is proportional to the significance of the overrepresentation of the term. From Genetic variation in putative regulatory loci controlling gene expression in breast cancer. Proc Natl Acad Sci U S A. 2006May 16;103(20):7735-40. Epub 2006 May 9. PMID:16684880 PMCID:PMC1458617DOI:10.1073/pnas.0601893103
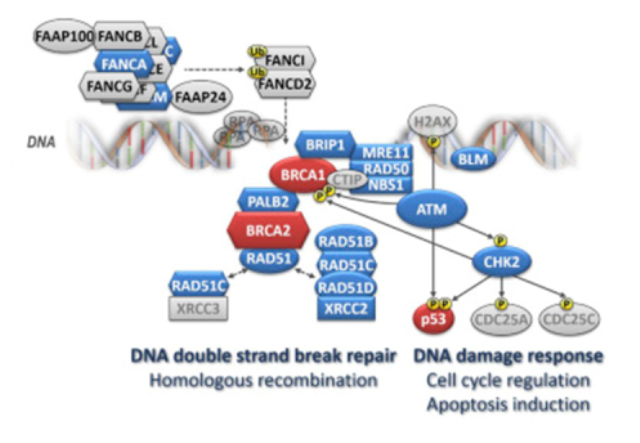
Figure 2. Schematic presentation of the involvement of currently established breast cancer-susceptibility genes' products in DNA repair and DNA damage response. Note: The major breast cancer-susceptibility proteins are colored in red, the other breast cancer-susceptibility proteins are in blue. Members of the large Fanconi’s anemia (FANC) family are depicted as hexagons. Several major rarely mutated breast cancer-susceptibility genes (PTEN, STK11, and CDH1) are not shown as they are not directly involved in the DNA repair and DNA damage response pathways. Homologous recombination (HR) is the most accurate DNA repair pathway resolving DNA double-strand breaks during the S – G2 phase of the cell cycle. This highly complex and multistep process requires a sister chromatid acting as a repair template. At the beginning, sensoric proteins that include the MRN complex (consisting of MRE11, RAD50, and NBN) bind to broken DNA ends and start a single-strand end resection (with the support of the BLM helicase) generating long single-strand overhangs. The MRN complex also contributes to the activation of the ATM kinase that in turn phosphorylates proteins (incl. CHK2 or p53) responsible for the orchestration of the DNA damage response (including cell cycle arrest or induction of apoptosis) and proteins (incl. BRCA1) involved in HR repair itself. The assembly of large nucleoprotein complexes for HR requires a proper spacio-temporal regulation. The BRCA1, BRCA2 proteins and other members of the Fanconi’s anemia (FANC-) protein family contribute to these processes. While BRCA1 facilitates numerous protein-protein interactions at the sites of the DNA break, the core complex, consisting of the FANCA-C, E-G, L, M, and associated FAAP proteins, is a multiprotein ubiquitin ligase activating FANCD2 and FANCI in response to DNA damage. When ubiquitinated, these proteins translocate to the DNA break site and contribute to the activation of HR with other downstream FANC protein family members, including BRIP1 (FANCJ), PALB2 (FANCN), and BRCA2 (FANCD1). Thereafter, the RAD51 recombinase, with the aid of several accessory proteins (“RAD51 paralogs”: RAD51B, RAD51C, RAD51D, XRCC2, and XRCC3), binds to the single-strand overhangs and promotes a search for a homologous sequence and its localization at the sister chromatid. From Women at high risk of breast cancer: Molecular characteristics, clinical presentation and management. Breast. 2016 Aug;28:136-44. doi: 10.1016/j.breast.2016.05.006. Review. PMID:27318168
Figure 3. Schematic distribution of breast cancer according to genetic risk. High-risk breast cancer patients recruit from a genetic breast cancer risk group in which the probability of breast cancer development gradually increases from the group of low penetrance alleles to the group of high penetrance genes (BRCA1, BRCA2, p53, CDH1, PTEN, STK11). The percentages are only approximate because the categories overlap, some borders are arbitrary, there are still uncharacterized genes/alleles in given categories, and the proportions of particular categories in the hereditary breast cancer risk group may vary in particular populations. From Women at high risk of breast cancer: Molecular characteristics, clinical presentation and management. Breast. 2016 Aug;28:136-44. doi: 10.1016/j.breast.2016.05.006. Review. PMID:27318168
Project members: Steffan Daniel Bos Haugen, Mev Dominguez Valentin, Grethe I. Grenaker Alnæs
PhD thesis from project:
1. Hege Edvardsen 09.05.2007; Title of the thesis: Genetic variation and response to radio- and chemotherapy and adverse side effects in cancer patients.
2. Silje Nordgard 15.05.2008; Title of the thesis: Genetic background and molecular phenotypes of breast cancer: From single gene variants to pathway and whole genome patterns.
External colaborators:
- Douglas Easton, and the BCAC consortium, Cambridge, UK http://ccge.medschl.cam.ac.uk/prof-douglas-easton/
- Olga Troyanskaya, Princeton University (NJ, USA) https://function.princeton.edu/
- The Kuijjer lab and The Mathelier lab at the Center for Molecular Medicine Norway (UiO) https://www.med.uio.no/ncmm/english/
- Kyriaki Michailidou, University of Central Lancashire https://www.uclancyprus.ac.cy/academic/dr-kyriaki-michailidou/
- Eivind Hovig, IKF, UiO https://www.ous-research.no/hovig/
Recent publications:
Breast. 2016 Aug;28:136-44. doi: 10.1016/j.breast.2016.05.006. Review. PMID:27318168
2. rs2735383, located at a microRNA binding site in the 3'UTR of NBS1, is not associated with breast cancer risk.
Sci Rep. 2016 Nov 15;6:36874. doi: 10.1038/srep36874. PMID:27845421
3. Genetic Risk Score Mendelian Randomization Shows that Obesity Measured as Body Mass Index, but not Waist:Hip Ratio, Is Causal for Endometrial Cancer.
Cancer Epidemiol Biomarkers Prev. 2016 Nov;25(11):1503-1510. PMID:27550749
4. Five endometrial cancer risk loci identified through genome-wide association analysis.
Nat Genet. 2016 Jun;48(6):667-74. doi: 10.1038/ng.3562. PMID:27135401
5. Fine-scale mapping of 8q24 locus identifies multiple independent risk variants for breast cancer.
Int J Cancer. 2016 Sep 15;139(6):1303-17. doi: 10.1002/ijc.30150. PMID:27087578
6. CYP19A1 fine-mapping and Mendelian randomization: estradiol is causal for endometrial cancer.
Endocr Relat Cancer. 2016 Feb;23(2):77-91. doi: 10.1530/ERC-15-0386. PMID:26574572
7. Comprehensive genetic assessment of the ESR1 locus identifies a risk region for endometrial cancer.
Endocr Relat Cancer. 2015 Oct;22(5):851-61. doi: 10.1530/ERC-15-0319. PMID:26330482
8. Height and Breast Cancer Risk: Evidence From Prospective Studies and Mendelian Randomization.
J Natl Cancer Inst. 2015 Aug 20;107(11). pii: djv219. doi: 10.1093/jnci/djv219. PMID:26296642
9. Novel Associations between Common Breast Cancer Susceptibility Variants and Risk-Predicting Mammographic Density Measures.
Cancer Res. 2015 Jun 15;75(12):2457-67. doi: 10.1158/0008-5472.CAN-14-2012. PMID:25862352
10. Assessment of variation in immunosuppressive pathway genes reveals TGFBR2 to be associated with prognosis of estrogen receptor-negative breast cancer after chemotherapy.
Breast Cancer Res. 2015 Feb 10;17:18. doi: 10.1186/s13058-015-0522-2. PMID:25849327
11. Genome-wide association analysis of more than 120,000 individuals identifies 15 new susceptibility loci for breast cancer.
Nat Genet. 2015 Apr;47(4):373-80. doi: 10.1038/ng.3242. PMID:25751625
12. Candidate locus analysis of the TERT-CLPTM1L cancer risk region on chromosome 5p15 identifies multiple independent variants associated with endometrial cancer risk.
Hum Genet. 2015 Feb;134(2):231-45. doi: 10.1007/s00439-014-1515-4. PMID:25487306
13. MicroRNA related polymorphisms and breast cancer risk.
PLoS One. 2014 Nov 12;9(11):e109973. doi: 10.1371/journal.pone.0109973. PMID:25390939
14. Fine-mapping of the HNF1B multicancer locus identifies candidate variants that mediate endometrial cancer risk.
Hum Mol Genet. 2015 Mar 1;24(5):1478-92. doi: 10.1093/hmg/ddu552. PMID:25378557
15. Evidence that breast cancer risk at the 2q35 locus is mediated through IGFBP5 regulation.
Nat Commun. 2014 Sep 23;4:4999. doi: 10.1038/ncomms5999. PMID:25248036
16.Genetic variation at CYP3A is associated with age at menarche and breast cancer risk: a case-control study.
Breast Cancer Res. 2014 May 26;16(3):R51. doi: 10.1186/bcr3662. PMID:24887515
17. The 5p12 breast cancer susceptibility locus affects MRPS30 expression in estrogen-receptor positive tumors.
Mol Oncol. 2014 Mar;8(2):273-84. doi: 10.1016/j.molonc.2013.11.008. PMID:24388359
18. Large-scale genotyping identifies 41 new loci associated with breast cancer risk.
Nat Genet. 2013 Apr;45(4):353-61, 361e1-2. doi: 10.1038/ng.2563. PMID:23535729
19. CHEK2*1100delC heterozygosity in women with breast cancer associated with early death, breast cancer-specific death, and increased risk of a second breast cancer.
J Clin Oncol. 2012 Dec 10;30(35):4308-16. doi: 10.1200/JCO.2012.42.7336. PMID:23109706
20. Common breast cancer susceptibility variants in LSP1 and RAD51L1 are associated with mammographic density measures that predict breast cancer risk.
Cancer Epidemiol Biomarkers Prev. 2012 Jul;21(7):1156-66. doi: 10.1158/1055-9965.EPI-12-0066. PMID:22454379
21. Genetic variation in putative regulatory loci controlling gene expression in breast cancer.
Proc Natl Acad Sci U S A. 2006May 16;103(20):7735-40. Epub 2006 May 9. PMID:16684880 PMCID:PMC1458617DOI:10.1073/pnas.0601893103
References:
- Fachal, L., Aschard, H., Beesley, J., Barnes, D.R., Allen, J., Kar, S., Pooley, K.A., Dennis, J., Michailidou, K., Turman, C., et al. (2020). Fine-mapping of 150 breast cancer risk regions identifies 191 likely target genes. Nature Genetics 52, 56-73. https://www.nature.com/articles/s41588-019-0537-1
- Chen, K.M., Wong, A.K., Troyanskaya, O.G., and Zhou, J. (2022). A sequence-based global map of regulatory activity for deciphering human genetics. Nat Genet 54, 940-949. https://www.nature.com/articles/s41588-022-01102-2
- Madireddy, L., Patsopoulos, N.A., Cotsapas, C., Bos, S.D., Beecham, A., McCauley, J., Kim, K., Jia, X.M., Santaniello, A., Caillier, S.J., et al. (2019). A systems biology approach uncovers cell-specific gene regulatory effects of genetic associations in multiple sclerosis. Nature Communications 10. https://pubmed.ncbi.nlm.nih.gov/31110181/