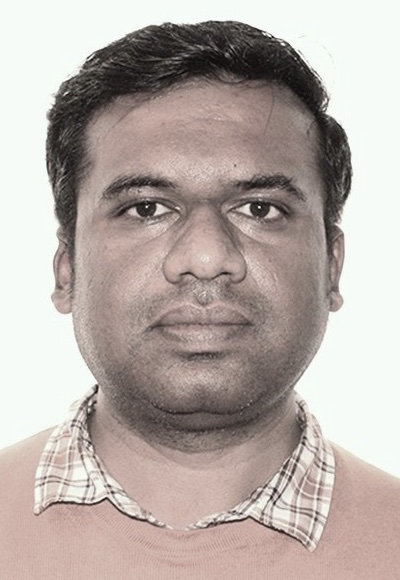
Naimahmed Nesaragi
- Post doc; PhD
About
Naimahmed Nesaragi is a Postdoctoral Researcher at the Technology and Innovation Clinic (The Intervention Centre), Oslo University Hospital. Naim obtained his Bachelor's degree in 2010 from Visvesvaraiya Technological University, Karnataka, India. Following this, he ventured industrial experience in automotive industry as an Associate Software Engineer for 2 years at Robert Bosch, India. Subsequently, he pursued his Master's degree at PES University Bangalore from 2013 to 2015, where he also undertook a Graduate Research Internship at CSIR-National Aerospace Laboratories (NAL) in Bangalore, contributing to his Master's Dissertation. Later, embarking on a trajectory of research, Naim joined as a Visiting Research Fellow at Raman Research Institute (RRI), Bangalore. In 2021, he earned his Ph.D. (Biomedical Signal Processing) from National Institute of Technology (NIT) Goa, India. His doctoral work titled “Modeling and Mining Schemes for Early Prediction of Sepsis using Higher-order Representations from Structured EHR data” delved into the intersection of Electronic Health Record (EHR) analysis and Physiological Signal Processing, employing Machine Learning for Critical Care Informatics.
Naim's primary research interests revolve around the classification and analysis of physiological time-series, particularly in the context of Intensive Care Unit (ICU) data, focusing on hemodynamic monitoring, cardiovascular diseases, sepsis and mortality. He has been actively participating in PhysioNet/ Computing in Cardiology (CinC) challenges hosted from 2016 to date, contributing open-source signal processing solutions/algorithms Notable research endeavors includes heart sound classification using phonocardiograms, classification of sleep arousals and stages using Polysomnography recordings, atrial fibrillation classification using ECG, neuro-prognostication in comatose patients using EEG etc.
During his postdoctoral stint, Naim has developed signal processing and machine learning algorithms to estimate early blood loss using vital signs for improved hemodynamic monitoring. Additionally, he is also involved in the analysis of accelerometer signals and pressure data to detect life-threatening complications in extracorporeal membrane oxygenation (ECMO) treatment.
Beyond his research pursuits, Naim is deeply passionate about fostering connections with fellow researchers and staying at the forefront of the latest advancements, particularly in the realm of Large Language Models (LLMs) and Generative AI. His current research interests are centered around the applications of diffusion models and prompt engineering, reflecting his commitment to exploring cutting-edge avenues in the field.
Research profile
Naimahmed Nesaragi’s research interest revolves around physiological signal processing, time-frequency analysis, higher-order decomposition schemes, computer-aided medical diagnosis, and critical-care informatics. He has authored over 10+ research papers as a first author in various reputed international journals/conferences: Crtical Care Medicine, Elsevier, IEEE Proceedings, IntechOpen, etc. Also served as a reviewer for prominent publications: Scientific Reports, Digital Health, PlosOne and IEEE-JBHI.
Links to personal pages at:
Publications 2025
Accelerometer-based vibration analysis and oxygenator thrombosis in venovenous ECMO: an experimental porcine model
Intensive Care Med Exp, 13 (1), 55
DOI 10.1186/s40635-025-00763-7, PubMed 40457022
Publications 2024
Electrical bioimpedance in the era of artificial intelligence
J Electr Bioimpedance, 15 (1), 1-3
DOI 10.2478/joeb-2024-0001, PubMed 38304720
Publications 2022
Automated diagnosis of coronary artery disease using scalogram-based tensor decomposition with heart rate signals
Med Eng Phys, 110, 103811
DOI 10.1016/j.medengphy.2022.103811, PubMed 35525698
Publications 2021
Tensor learning of pointwise mutual information from EHR data for early prediction of sepsis
Comput Biol Med, 134, 104430
DOI 10.1016/j.compbiomed.2021.104430, PubMed 33991856
Publications 2020
Early Prediction of Sepsis From Clinical Data Using Ratio and Power-Based Features
Crit Care Med, 48 (12), e1343-e1349
DOI 10.1097/CCM.0000000000004691, PubMed 33048903