Protein Expression
Dysregulation of different genes is known to contribute to cancer development through several different mechanisms. Divergent levels of a protein may provide information relevant to diagnosis, prognosis or treatment decisions for patients.
We seek to gain further knowledge about the relationship between protein expression and the development of cancer, to increase our mechanistic insight and identify possible prognostic markers for cancer patients. Immunohistochemistry (IHC) is one of the most widely used diagnostic procedures in modern medicine and is used to determine the expression levels and subcellular localisations of specific proteins in a tissue sample.
We are currently conducting IHC studies of a panel of selected proteins involved in relevant processes such as cell cycle regulation checkpoint and genomic instability. We have already identified a large number of potential cancer-related proteins in sections from patients with prostate carcinomas. We examine the relationship between the protein expression and clinicopathological variables, including patient survival, with the main goal of identifying prognostic biomarkers for patients with these cancer types and exploring their mechanisms.
In Immunohistochemistry, we use antibodies that recognise and bind to a specific region within the protein of interest. Different methods can be used for visualisation of this bound antibody. In the direct method, the antibody is conjugated to a reagent, e.g., an enzyme, that catalyses a colour-producing reaction or a fluorescent tag. The indirect visualisation system uses an enzyme-conjugated secondary antibody, which binds to an unlabelled primary to amplify the signal. The enzyme reacts with a chromogen substrate such as 3, 3’-Diaminobenzidine (DAB) or 3-Amino-9-ethyl carbazole (AEC), producing a brown or red product, displaying where the targeted protein is present. The chromogenic signal can be amplified using different methods. We use the polymer-based detection method, where antibodies and enzymes are conjugated to a polymer backbone, enhancing the sensitivity and specificity of the signal.
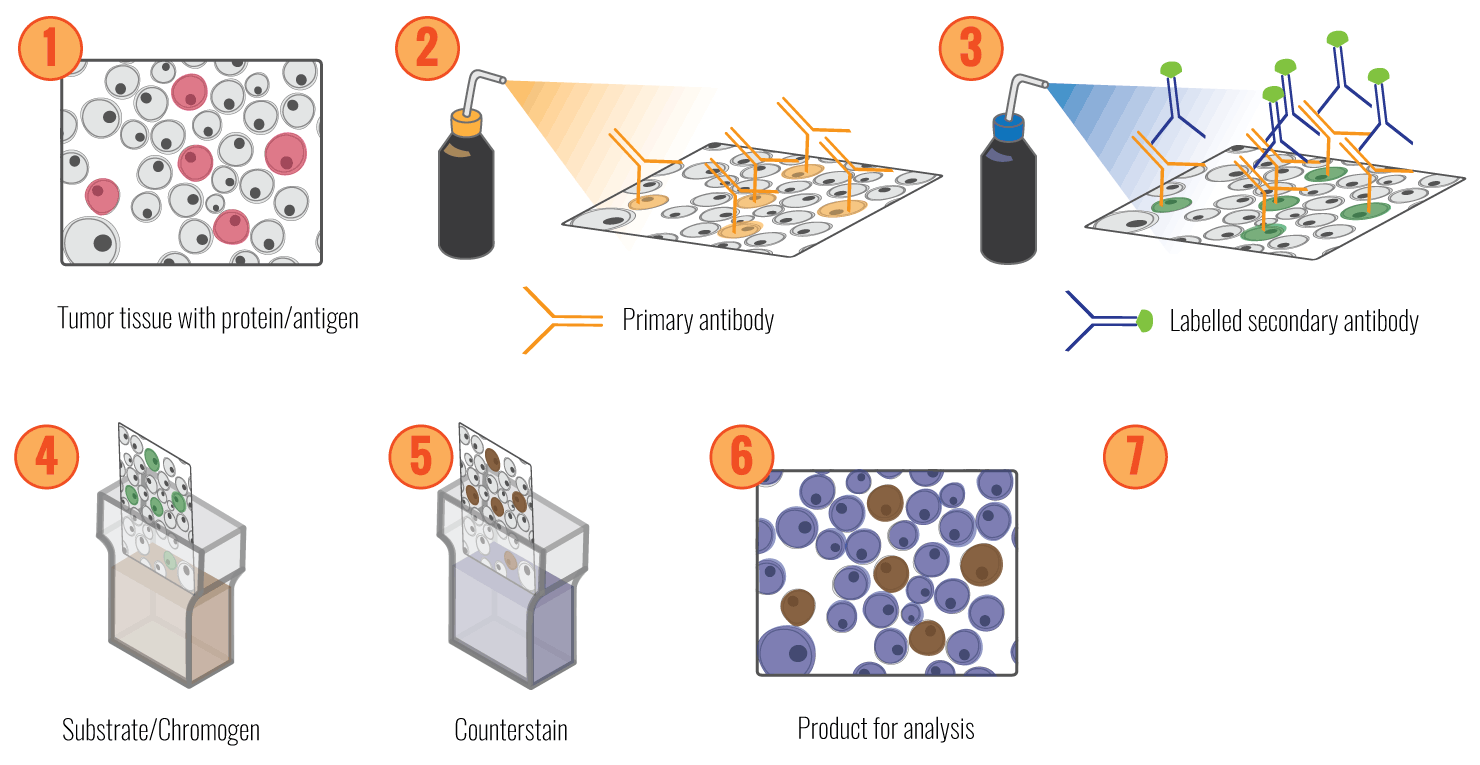
As the scoring of positive and negative cells after IHC is both laborious and in some instances quite challenging for humans, we have developed a machine learning system (ImmunoPath) to automatically and reproducible score IHC samples. A part of this project is to evaluate and further develop this system.
Automated detection and scoring of Protein Expression
Automated detection and scoring of protein expression detected by Immunohistochemistry are preferable to the manual scoring routinely used today, an imprecise and time-consuming process. Using Immunohistochemistry, divergent levels of a protein may provide relevant information for the diagnosis, prognosis, or treatment decisions for patients. Considerable experience is required to interpret the staining patterns produced through IHC, and the visual evaluation of immune stained tissue sections is time-consuming for pathologists. Furthermore, the method is challenged by variance among and between observers.
We created ImmunoPath, an image system based on traditional machine learning algorithms, to suit the need for an efficient, automated, objective, and reproducible system. ImmunoPath was developed in collaboration with pathologists, medical researchers, and digital software developers. The interdisciplinary cooperation has resulted in an application for visualisation and quantification of sections stained with various antibodies. The accuracy of the automatic quantification depends on the staining pattern, which varies according to the antibody clone, staining procedure and the protein and tissue under investigation. Proteins that produce a strong stain in a low percentage of the cells, such as cyclin B1 (CCNB1) or PTTG1 regulator of sister chromatid separation, securin, are accurately quantitated by ImmunoPath. Other staining patterns, such as those of phosphatase and tensin homolog (PTEN) or BUB3 mitotic checkpoint protein, have proven more challenging and require further algorithms. We are, therefore, currently working on implementing artificial intelligence in the form of deep learning algorithms to score proteins with more diffuse staining patterns, such as PTEN and BUB3. Learning sets will have to be established by manually identifying and segment a large number of positive and negative cells, allowing the neural nets to learn how to detect the difference. Preliminary results indicate that markers scored by ImmunoPath have a stronger prognostic power than results after manual scoring:
Additionally, we are focusing on establishing a procedure to evaluate the accuracy of specific immune scoring protocols. This method will help us to choose an optimal protocol for proteins that can be scored with the conventional ImmunoPath, and decide which proteins should be scored with algorithms that use deep learning.
The evaluation of a set of proteins frequently used in clinical practice will be performed in cancer tissues (prostate, breast, CRC and lung) and used to describe the strength and limits of the system in a forthcoming paper, where the automated scoring will be compared against manual scoring and prognostic power.
Protein expression of candidate genes in prostate carcinomas
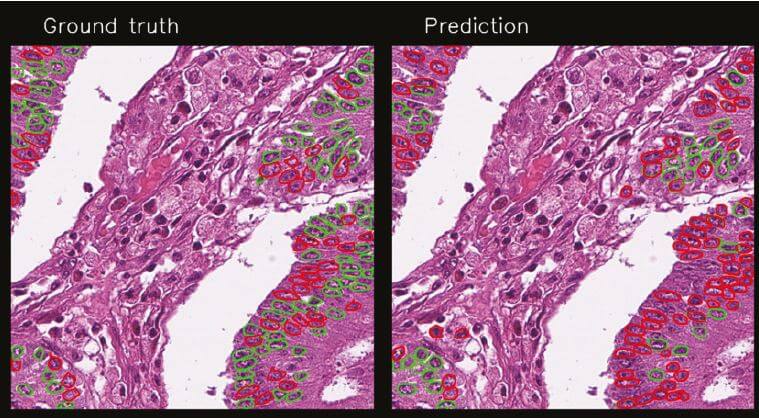
This project began several years ago, based on a selection of 71 candidate genes with an expected role in prostate cancer: Twelve of 38 proteins (32%) scored and analysed are shown to be significantly linked to disease outcome in univariate analysis of time to recurrence (TTR) and hence, are assumed to play a role in prostate cancer. The remaining 33 proteins are currently being scored and analysed.
Based on the preliminary results, we expect to identify 23 prostate cancer-related proteins among our candidates, and they will all be evaluated as potential biomarkers of prognosis. We hope to get a further understanding of the mechanism behind each protein’s relation to prostate cancer by studying their interactions and functions.
Pseudo-IHC; scoring of Ki67 in HE-stained sections
A new concept for training deep learning networks has recently been introduced at the institute. We are staining tissue sections with HE for routine diagnostics, scanning the section, removing the stain and then re-staining the section using IHC. In this way, we can identify cells of interest, transferring the identification to the same cells in the HE-stained section and use this identification to train a deep learning network to recognise the cells of interest directly in the routine diagnostic section. If we succeed in developing an algorithm for a given cell or cellular feature, they can be automatically scored in routine diagnostic sections. Early results with Ki67 were very promising, but there was room for improvement. Through more clearly identifying the cells expressing Ki67 by drawing around them, a new training set was created from 900 tiles (800x800 pixels) selected randomly from 100 patient samples, and all positive and negative epithelial tumour cell nuclei were drawn around in 308 image tiles using proprietary software (Nline), resulting in 38 715 scored objects. These were used to train a Mask R-CNN model.
The new training set gave improved results, which are very promising, but there are still both false positives and false negative cells. Hence, there is still room for improvement of the algorithm. We see that some images do particularly better than others and that both morphology and staining influence the results. The next steps entail:
- Augmentation to introduce more variation in the training set to make the network more invariant to staining
- Optimising the training set by using only distinct cells
- Increase the size of the training set
- Evaluate other models
Automated scoring of PD-L1
Immunotherapies targeted against programmed death-ligand 1 (PD-L1) and its receptor (PD-1) have improved survival in a subset of patients with advanced lung cancer. PD-L1 protein expression has emerged as a biomarker that predicts which patients are more likely to respond to immunotherapy. The understanding of PD-L1 as a biomarker is complicated by the history of use of different immunohistochemistry platforms with different PD-L1 antibodies, scoring systems, and positivity cut-offs for immunotherapy clinical trials with different anti-PD-L1 and anti-PD-1 drugs. ICGI was challenged by MSD, who produce Keytruda (Pembrolizumab) immunotherapy drug against PD-L1, to use deep learning to develop an automated scoring system. We accepted the challenge, and through the pathology departments at OUS and UCL we gained access to close to 900 IHC cases stained with an antibody against PD-L1, as well as the pathology scores for each case. Training and test sets were designed from about 100 cases by drawing and scoring cells, and the preliminary results are very promising.
Overall results are very good, but there are issues with false positives and false negatives that make the scorings around the 1% threshold (a clinical threshold for administering the immunotherapy) less reliable, and this must be solved before the method can be used in clinical diagnostics. The next steps can entail:
- Improving the network by including a model that excludes non-tumour areas
- Optimising the training set by including distinct cells only
- Extend the training set