Histotyping
One of the products emerging from the DoMore! project is called “Histotyping”, which uses the marker DoMore-v1-CRC, and culminated in 2020 in a landmark artificial intelligence (AI) publication in the Lancet (Skrede et al., The Lancet 2020).
Histotyping involves an automated analysis of high-resolution scans of cancerous tissue stained with routine hematoxylin and eosin (H&E). ICGI developed convolutional neural networks for this method and trained on extensive retrospective clinical trial data with known patient outcomes. Deep learning models were trained to identify and analyse the morphology of a tumour in a surgically resected tissue section. Histotyping estimates the probability of a good or poor outcome, i.e., the likelihood of a recurrence. It could be employed as a supplementary diagnostic service to support clinicians and patients in their adjuvant chemotherapy decision-making for patients
with stages II and III CRC.
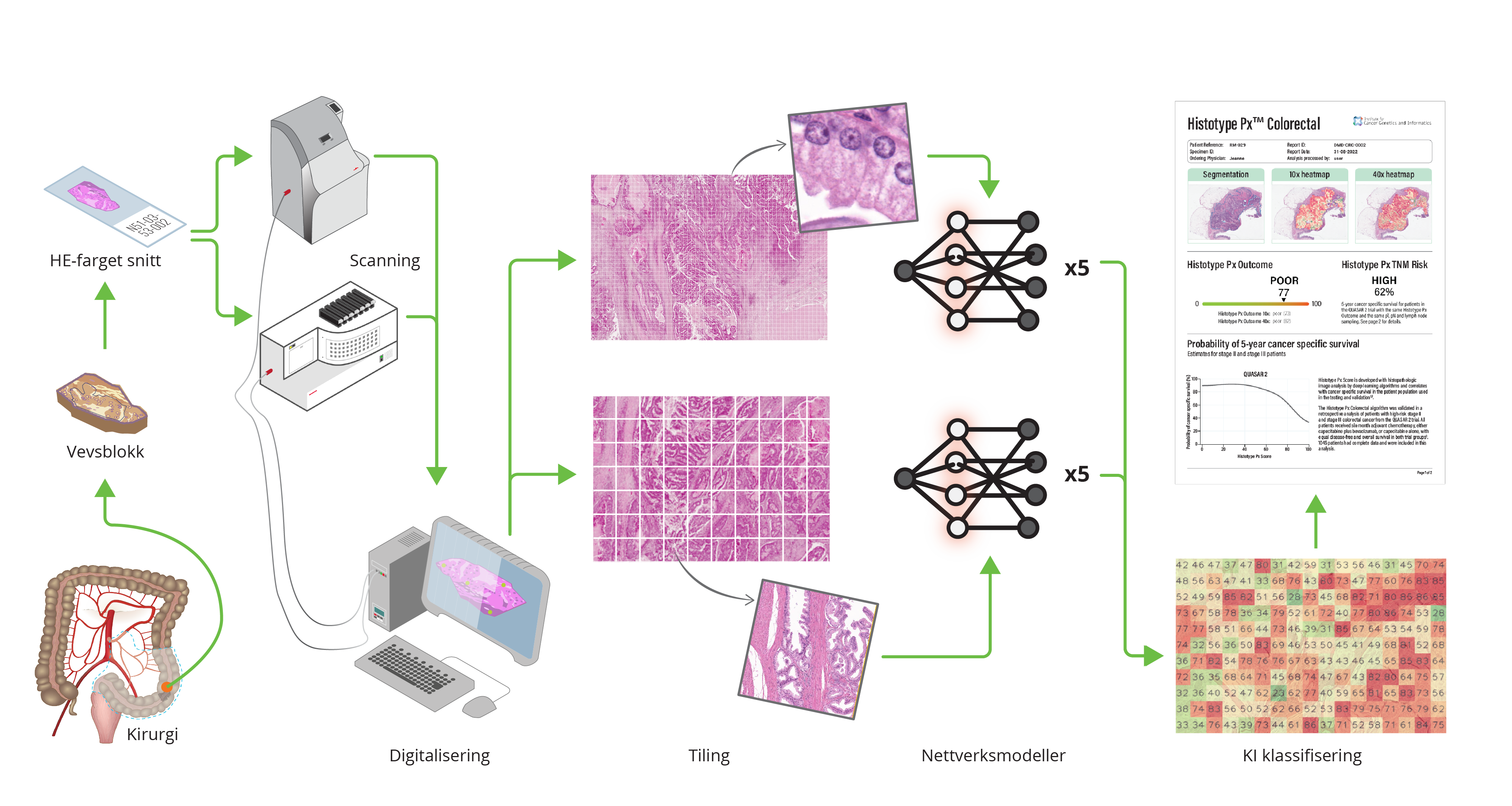
The DoMore-v1-CRC marker has also been combined with established clinical pathology markers (T and N stage, and number of harvested lymph nodes) in a decision tree solution for clinical decision support. Compared to conventional risk stratification for adjuvant therapy, the proposed method identified a significantly larger group of patients with a good prognosis that are candidates to be spared adjuvant treatment (Kleppe et al., Lancet Oncology 2022)
Using the DoMore-v1 network, a similar approach has been performed on prostate cancer patients. The adaption of this method from CRC to PCa included using sections from multiple tissue blocks as input images, as prostate tumours are far more heterogeneous than colorectal tumours. The product, the DoMore-v1-PCa marker, predicts the risk of recurrence after radical prostatectomy, and the marker shows strong prognostic results (HR=7.6) when tested in a publicly available cohort (TCGA-PRAD). Final validation and publication has been submitted.
Initial results in non-small cell lung cancers also show comparable results, and the project is expected to be completed by 2023. With the increasing number of publications on deep learning and systems claiming
to perform comparable with or better than clinicians, few have yet to demonstrate real-world medical utility. We published a Perspective in Nature review Cancer in 2021 to discuss the reasons for the moderate progress and to present our suggestions for recommended protocol items for the field to facilitate the transition to the clinic (Kleppe et al., Nat Rev Cancer 2021).
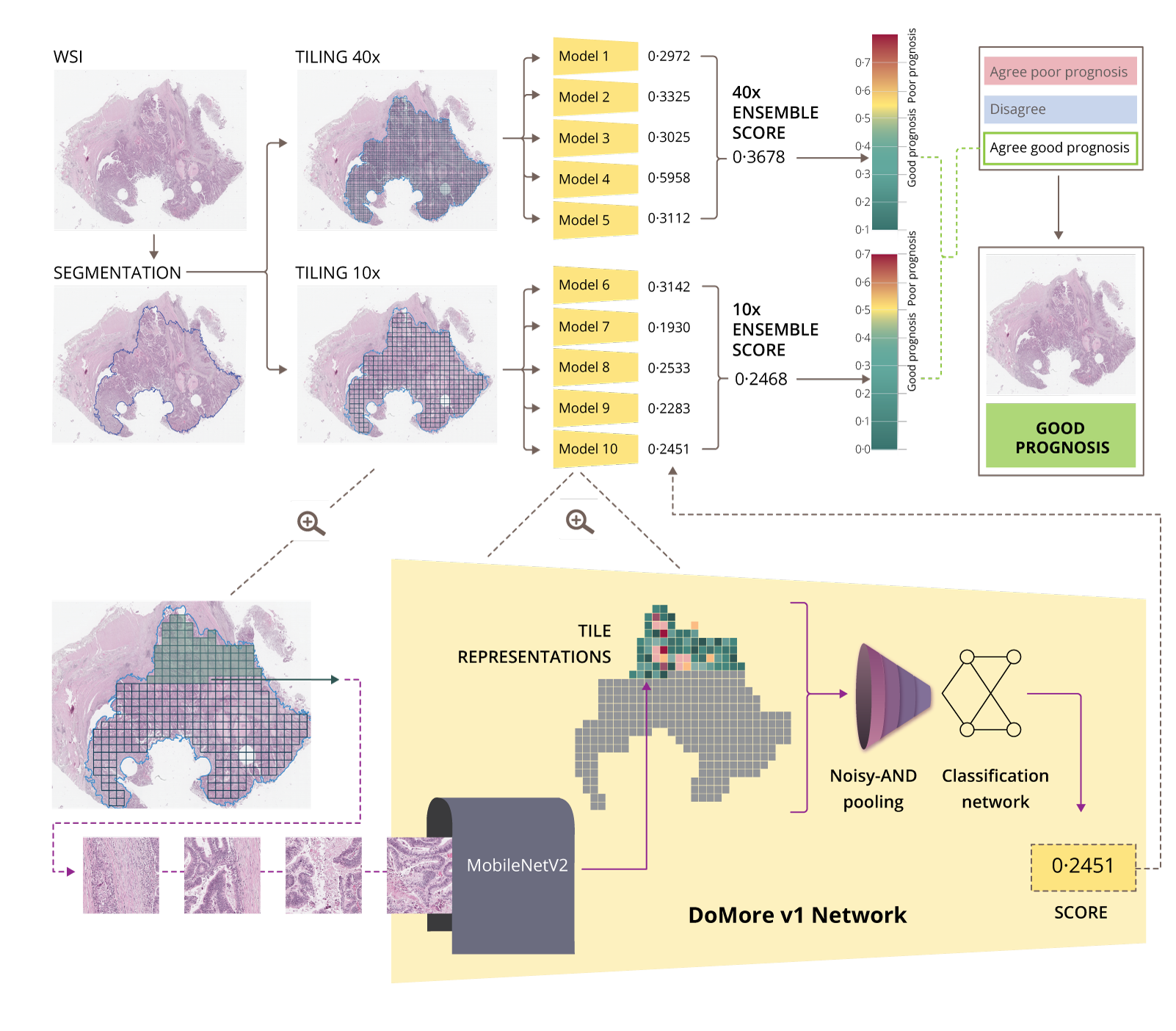