Learning from Deep Learning
Deep learning allows computers to do what comes naturally to humans: learn by example. Increasing knowledge of the biological basis for the predictions made by computerized systems is essential for implementation in the clinic.
We aim to gain an understanding of the systems, make them easier to use in conjunction with other pathological, biochemical, and clinical information, and help understand the mechanisms underlying metastatic disease.
With enough data and well-designed experiments, artificial intelligence (AI) systems clearly outperform competing techniques in areas such as image analysis, and a rapidly increasing number of publications now demonstrate the high performance of convolutional neural networks (CNNs) in medical diagnostics. With the anticipated large turnout of digital pathology, AI systems are expected to play a rapidly increasing role, including the prediction tasks based on histopathological images.
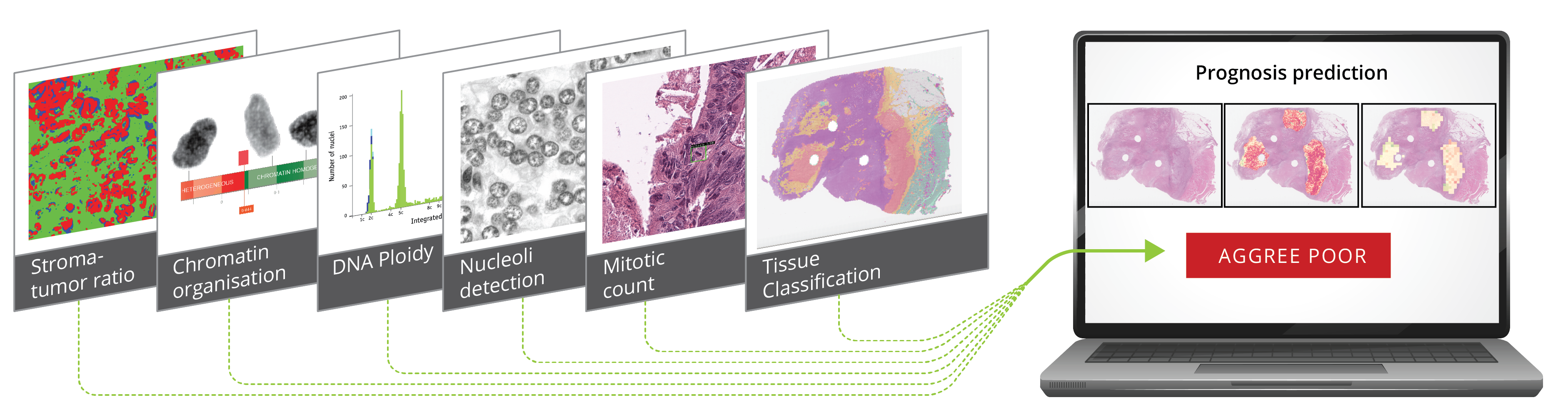
Improved cancer relapse predictions may help avoid cytotoxic therapy in low-risk groups and identify patients who might benefit from intensive treatment regimes. However, few AI-based systems have yet reached the clinic, a primary concern being the "black box" nature of the systems. When the basis of the predictions is not traceable by humans, it also prohibits using the CNNs as tools in studies of biological factors and underlying disease progression.
We acknowledge the need to improve digital pathological images, such as changing or modifying staining to better display entities expected to impact prognosis, potentially leading to increased prediction performance. Recent developments have provided methods that enhance our ability to detect and visualize image areas of particular importance to network predictions. The need to supplement the information in the standard histopathological Haematoxylin and Eosin-stained images (HE-images) with concrete biomedical information, including measurements on a cellular level such as mRNA, DNA, chromatin organization, protein expression, and presence of mitotic cells is essential. The project builds on the competencies acquired over the years in the combined biomedical and informatics milieu at the Institute of Cancer Genetics and Informatics (ICGI) and in the large-scale DoMore! Lighthouse project, further strengthened by extended participation from the machine learning section at the Department of Informatics, University of Oslo (IFI).
The commercially available scanners introduce quality tradeoffs to make speed and image size more manageable in the diagnostic environment. These tradeoffs are acceptable for visual evaluation but may limit the potential of image analysis. ICGI presented the first WSI in 1996 and prototyped one of the first scanners on the market (Hamamatsu Nanozoomer). Our latest contribution is a scanner tailor-made to generate high-fidelity images for AI with oil immersion optics and an addressable illumination spectrum. A direct optical fiber connection from the camera to a high-performance cluster reduces the scan time of a larger number of pixels. In addition to the fast network, high field number optics, and real-time GPU analysis, our robotic immersion, handling, and tracking system cuts the person-hours needed to execute this part of the project. Right: HE section scanned at maximum resolution (40x, na=0.7) with the Aperio AT2 scanner. Left: The same section scanned at maximum resolution (60x, na=1.4) with our proprietary HighRes scanner. Images in yellow at maximum tolerated magnification.
Learning from deep learning is a project enabled by findings in the DoMore! project